This article is a guest post by Paramu Kurumathur.
[Pramu has over 37 years of industry experience and is an expert in Project / Program Management and Project Health. Paramu is a principal consultant with PM Power Consulting and the practice lead for PM Power’s Strategic IT suite of services and Program Management suite of services.]
(This article is an edited extract from PM Power’s upcoming book Full Stack Leadership. The original blog can be found here.)
It is interesting to see where AI has reached from when it started. Research on AI started as early as the 1950s and 1960s. By the beginning of this millennium, newer AI models like deep neural networks and ways to manipulate them, replaced the earlier models like Bayesian networks of the 1980s and 1990s, which had replaced the still earlier models like Markov chains. These new models of representing, storing, and retrieving knowledge are what drive the neural networks, transformers, natural language processing, language models and learning that make up generative AI. In addition, the explosion in chip technologies with organizations like Nvidia making huge strides in areas like GPU and TPU, also helps.
The field of AI keeps galloping ahead and is now manifesting itself as generative AI, which can create data on its own, rather than just read and interpret data supplied to it. AI can now make content, pictures, videos, and also support scientific research. Large data and AI together can produce unbelievable results with the AI machines spotting patterns, styles, and structures in the data they see. When it has learned from these data sets, it can make new content all on its own. I have even heard and played music made by AI. AI can bring in more productivity and efficiency, allow for better decision-making, help in experiments, and innovation and accessibility.
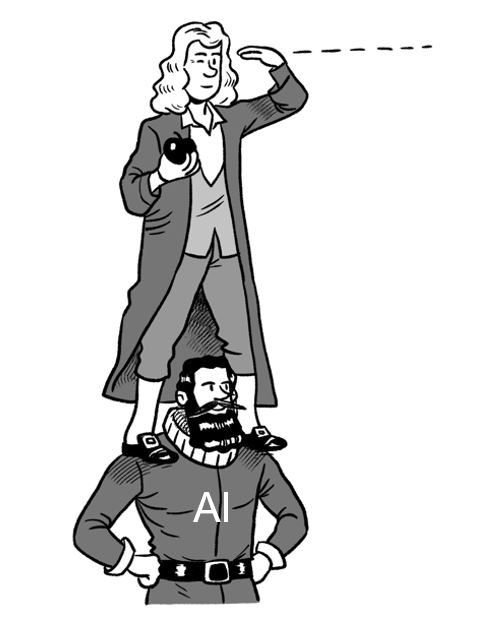
Of course, with its vast potential, it also brings great dangers like job loss, information bias, privacy issues, transparency issues and so on. And it does raise serious ethical concerns and worries that it will cause harm to society. AI could be used in the wrong way to hurt people and societies.
Some of the ways these dangers can be mitigated are developing internationally recognized guidelines on the use of AI, addressing biases and lack of transparency, and investing in awareness of the benefits and dangers of AI.
While we cannot get into details, let us quickly go over some of the things that leaders can do to ensure that AI is used and harnessed to serve organizations, communities and the world in general.
All principles of leadership will be affected one way or another by AI. But let us focus on those that are most affected.
- Looking for opportunities to grab them
- Breaking tradition and finding trailblazing ideas
- Data driven decision making
- Active inertia
- Need for high level of emotional quotient
- Protection against cyberattacks
- Trustworthiness
A true full stack leader will always be looking out for opportunities to grab them and will always be ready to break tradition and find trailblazing ideas. AI is creating, and will continue to create, a rapid change in the world of technologies and applications. This makes it important that we change the way we look for new ideas. What we have now is to be considered ‘tradition’. Also, AI will throw up new opportunities for us to grab. These new inputs will also change the way experimentation is done.
Another principle that needs to be looked at in the light of AI is data-driven decision making. AI can be used with great advantage to generate data, and also analyse it. But, if we are making decisions based on this data, we need to ensure the correctness of the data. AI can build biases into the output it gives based on what information it has access to. It can also generate fake data inadvertently or even maliciously. We can also be overwhelmed by the amount of data produced by AI.
Yet another aspect that the new avatars of AI can help with is dealing with active inertia. They can be used to study current systems and suggest better and more efficient processes and systems. Knowing human nature, changes suggested by a machine may be more acceptable that changes suggested by humans! Using the same techniques, AI can also help in supporting principles policies like ‘no waste’.
One of the key requirements of a good full stack leader is to have a high level of Emotional Quotient (EQ). One of the main worries that AI brings in is the loss of human connection. Human connections are essential even when we allow AI to take over many jobs. You may find that this human connection is slipping away with the taking over by AI.
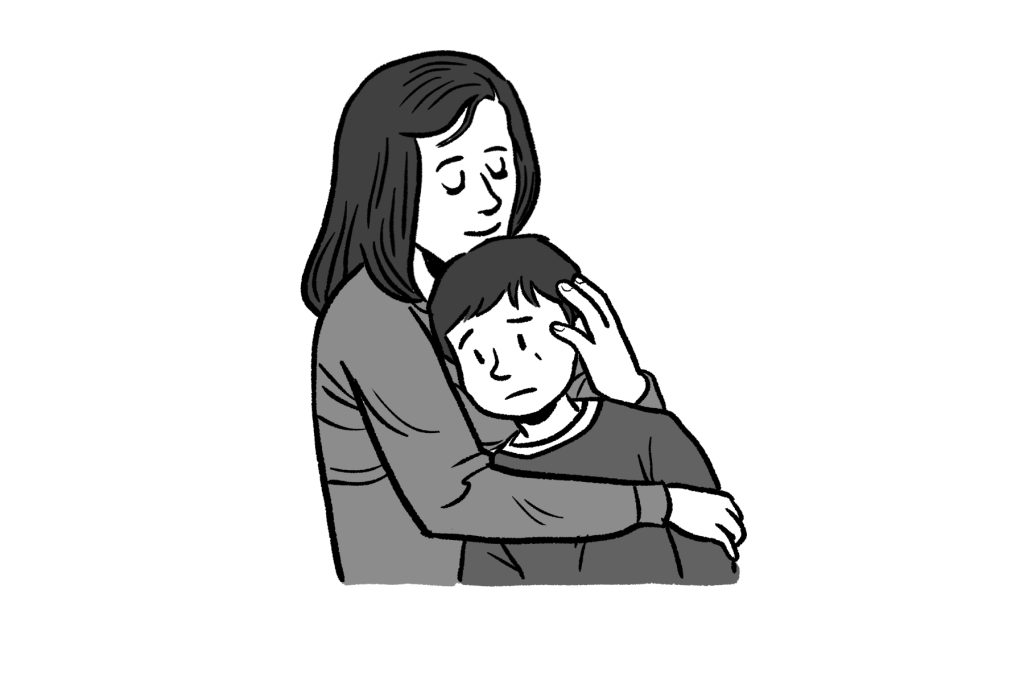
AI may not show the same empathy that humans do. Leaders must ensure that this does not happen. They must develop empathy and foster a culture of developing empathy and building strong relationships in the AI age. Also, interacting with AI driven systems continuously may affect associate well being and tolerance levels. Leaders must prioritize mental health and ensure associates have the support they need to work without stress.
Trustworthiness is another area that gets affected. Leadership is majorly about trust and inspiration. Even as humans, we are often challenged by these two facets. They are major levers for motivating individuals and teams. Some of the issues coming from partnership with AI like job displacement, bias and discrimination, lack of empathy, making decisions based on fake information can affect a leader’s trustworthiness. If these issues can be addressed, leaders can harness the potential of AI to enhance their trustworthiness and effectiveness.
Another aspect of AI that leaders look to take advantage of is protection against cyberattacks. AI can help process vast amounts of data, learn from patterns, and quickly detect and thus prevent threats. Leaders must be smart enough to ensure the harnessing of AI power to detect and prevent attacks. Of course, the same technology will be used by criminals to perpetrate cyber attacks. But a good leader should stay one step ahead of criminals.
One area that, though not mentioned as a principle in the book, has seen some new challenges that need to be addressed is ethical considerations, as we mentioned before. We saw some of the factors of this above when we discussed affected principles. Some of the other factors are:
- AI generated information can be used for copyright infringement.
- Deep fake information created by AI can be used to spread misinformation and lies about people to their detriment. It can also be used to manipulate people for the perpetrator’s benefit
- Accountability: AI can be blamed for mistakes made by people.
- AI based surveillance and analysis can be used to infringe on people’s privacy.
- The question whether AI can employed to replace humans in jobs, thus depriving people of livelihood, is also a serious concern.
Human tendency is very inventive, especially when it comes to circumventing aspects of ethics, despite compliance requirements that constantly keep increasing. That’s because, deep down, matters of ethics (or otherwise) are in the realm of the subconscious and culture. This is an area where AI can at best come up with insights, hypotheses, point at vulnerabilities but never be good enough as humans in terms of morally correct thinking and actions.
In summary, I would stress the word “Synergy” between Humans and AI, for leveraging each other’s capabilities and keeping our sanity close to our sleeves to ensure goodness.
[Thank you, JV, for editing and correcting this piece. Thank you, Srini and Mohan, for suggesting improvements.]